Digital endpoints and patient peported outcomes
The widespread use of commercially available wearable devices has unlocked the use of critical digital endpoints like heart rate, physical activity, and sleep data in clinical research. Each of these sensor measurements have a well-known connection to general health—increased physical activity, lower resting heart rate, and proper sleep typically lead to a longer, healthier life. In addition to general health, heart rate, physical activity, and sleep have a demonstrated connection to numerous patient reported outcomes. Physical activity has been linked to the prevention of premature death and chronic diseases like cardiovascular disease, diabetes, cancer, hypertension, obesity, and osteoporosis. It has also been shown to reduce symptoms of depression, anxiety, and panic. These factors have led to physical activity’s frequent use as an interventional tool in clinical research. Similarly, heart rate is considered a key indicator of cardiovascular events like heart failure, stroke, and renal failure. Its derived measurement, heart rate variability, is a clinically relevant diagnostic metric, widely used to represent autonomic nervous system function. Finally, insufficient sleep is connected to cardiovascular health, metabolic health, mental health, immunologic health, human performance, cancer, pain, and mortality.
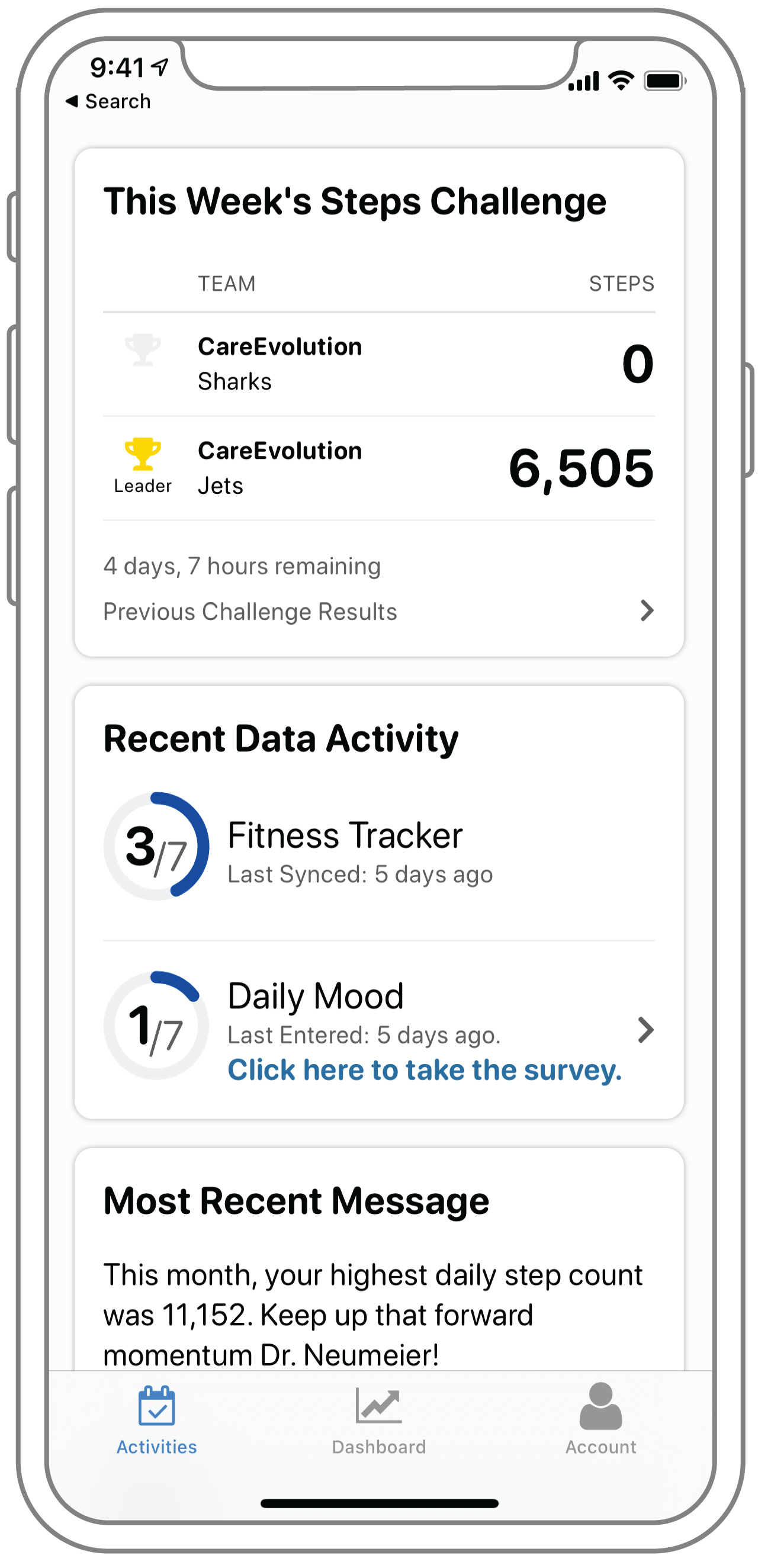
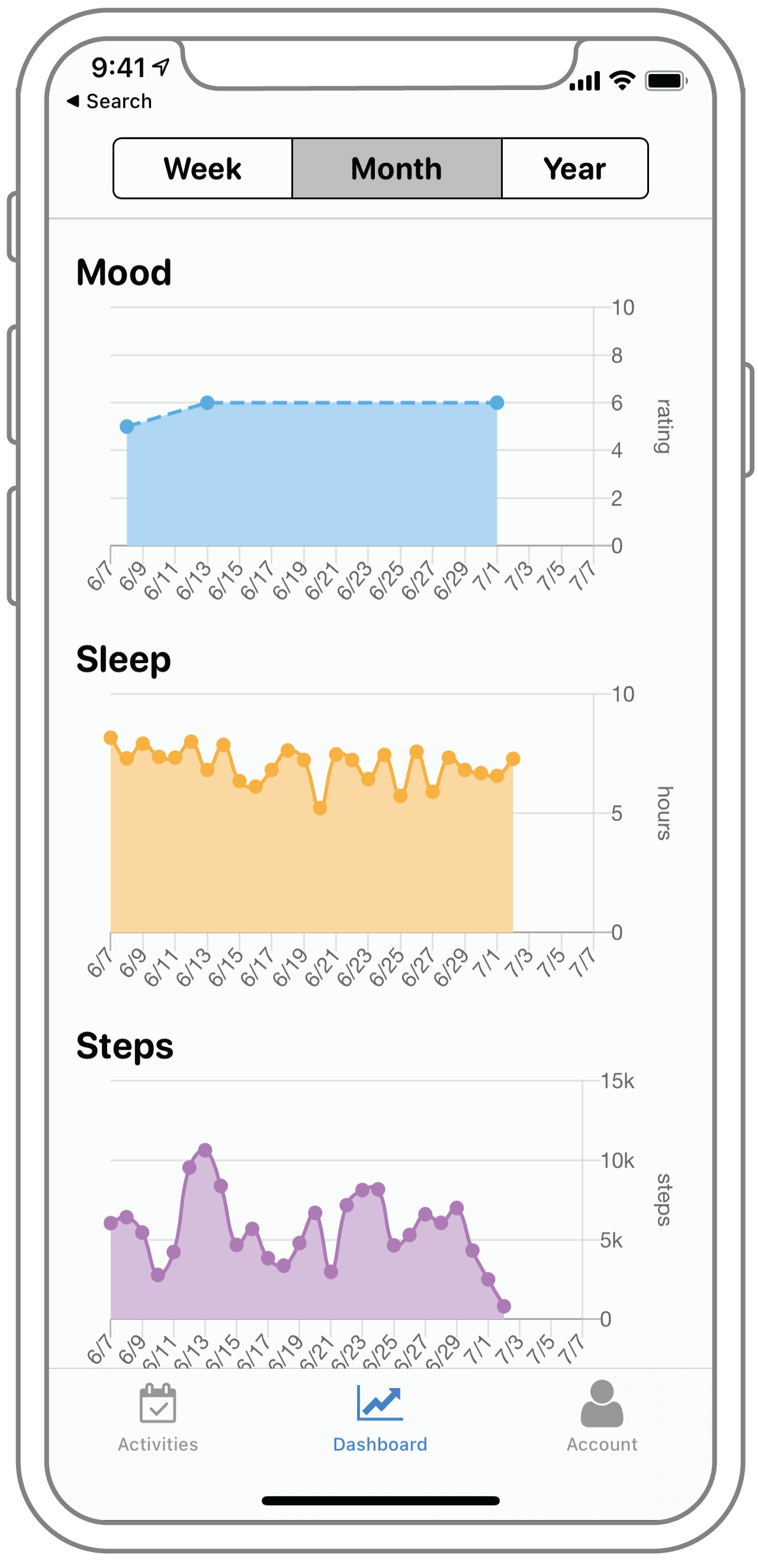
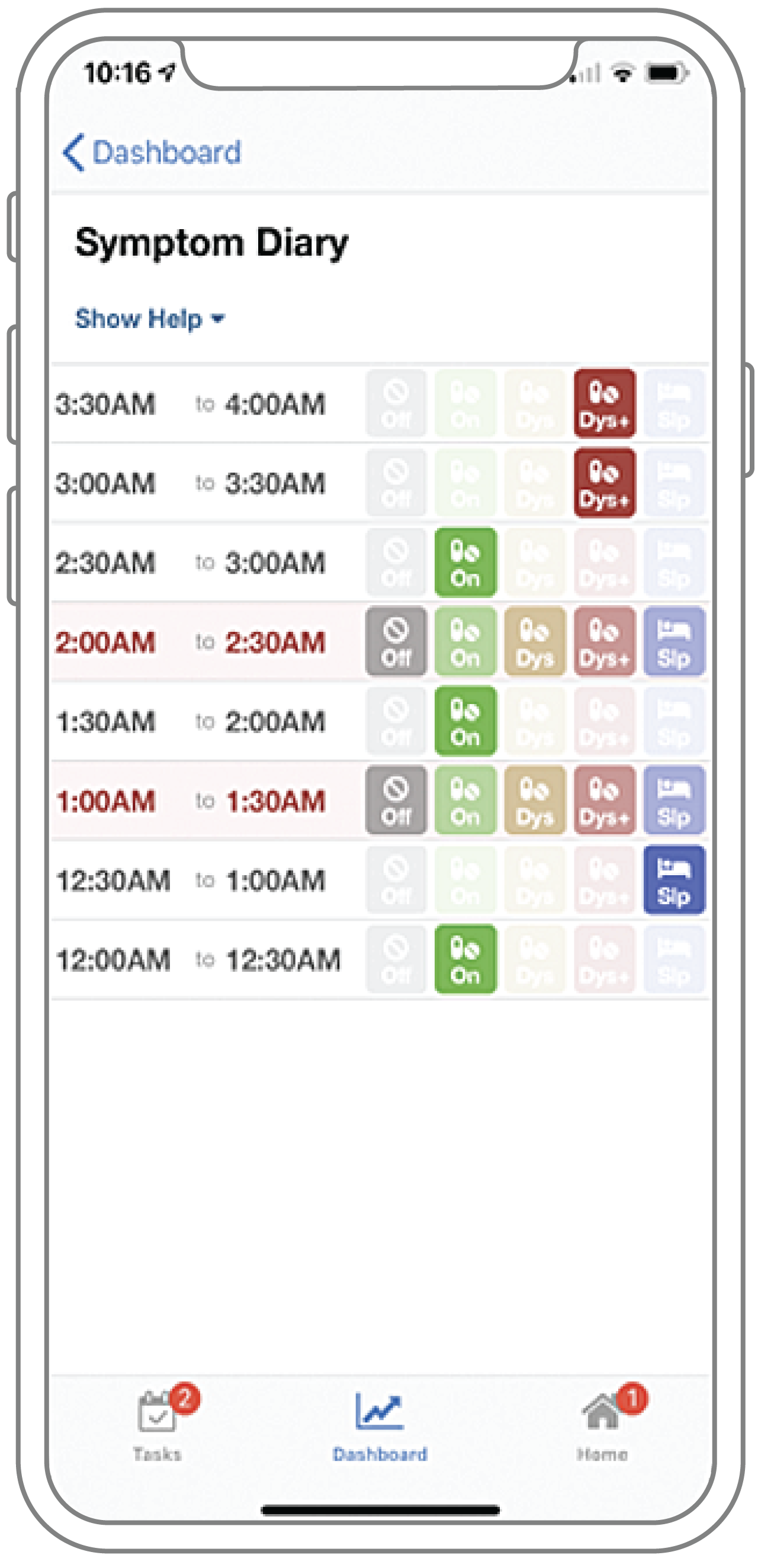
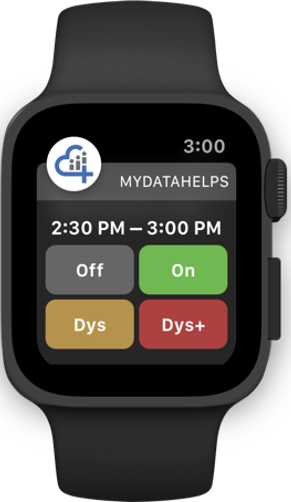
Given the remarkable applicability of these digital endpoints, consumer-directed wearable devices like Fitbit, Apple Health, and Google Fit are frequently incorporated in clinical research. The recent adoption of digital health platforms, like CareEvolution’s MyDataHelps™ digital clinical trial and research platform, has been an important driver of this incorporation. The collection of sensor data with MyDataHelps™ is easy, enabled by a simple checkbox in each project’s settings, and the data is seamlessly combined with survey results, electronic health records, and more in one comprehensive data export. This easy facilitation highlights the potential of including heart rate, physical activity, and sleep data in your next digital clinical research study.
Wearable device data in clinical research
The ubiquity of wearable devices by consumers indicates that their adoption for digital clinical research holds great potential in a diverse set of fields.
Pain
Sensor data provides an objective measure of function, which is an important outcome measure of pain and stiffness. The University of Michigan’s Opioid Prescribing Engagement Network (OPEN) utilizes wearable digital endpoints in their mission to develop recommendations for pain management to help prevent chronic opioid use.
Cardiology
Clinical wearable devices are historically used in the cardiology field to monitor heart rate, rhythm, and more. The adoption and validation of consumer-directed wearable devices are thus a natural extension of cardiology clinical research, facilitated by digital health platforms. For example, the Framingham Heart Study, launched in 1948, is a longitudinal cohort study investigating what factors impact cardiovascular health. In 2016, the study expanded to include an electronic cohort that uses the MyDataHelps™ mobile health app to complete surveys, perform weekly blood pressure measurements, and gather Apple Watch data.
Mental health and wellness
The impact of physical activity and sleep on mental health and wellbeing leaves wearable devices poised to play a critical role in digital clinical research. The Providing Mental Health Precision Treatment (PROMPT) study combines wearable data with DNA, wellness apps like Headspace, and survey data to provide a holistic digital phenotype with the ultimate goal of improving mental health care through increased access to care and better matching to treatment.
Precision medicine
The depth of data produced by wearable devices opens the door to precision medicine research. Let’s take the All of Us Research Program and Prediction of Glycemic Response Study (PROGRESS) as two remarkable examples of how sensor data are integral to precision medicine. The All of Us Research Program is a disease agnostic observational cohort study, gathering an unprecedented amount of data on diverse populations to facilitate precision medicine research. Heart rate, physical activity, sleep, and other device data can therefore be combined with data on a range of topics, including social determinants of health, family health history, and health care access, to answer new exciting research questions. More specifically, in the area of precision nutrition, PROGRESS combines wearable data with electronic health records, biosamples, and patient generated data to identify what influences how your body responds to food.
Epidemiology
In the field of epidemiology, wearable devices can provide useful data for clinical research. Consider the DETECT study, which investigates whether sensor data like heart rate can identify COVID-19 positivity when combined with symptom tracking. The digital endpoints that indicate viral infection can be subtle and are only possible because of the access to a steady stream of data provided by wearable devices—in this case connected to the MyDataHelps™ digital clinical trial and research platform.